This article describes how behavioral science can be applied in cardiometabolic clinical research to augment drug interventions. This combined approach addresses the Health Inequity gap while also increasing patient engagement.
Clinical Scenario Case Vignette
Years ago, one of us was a young doctor in conversation with a patient regarding enrollment in a Type 2 Diabetes research study. The conversation related to the patient’s high use of basal insulin (>150U/day) and dietary habits around healthy eating. The patient explained that he lived alone and his daughter had repeatedly tried to make him eat healthier by introducing vegetables as a dominant part of his diet, but he couldn’t tolerate the change. He became uncomfortable, dizzy, sweating, fatigued, and hungry – all classical symptoms of hypoglycemia. He stopped the healthy diet and resumed his normal diet. When asked how he had managed to reduce his insulin dose with the healthy diet to anticipate the lower amounts of carbohydrates, he looked baffled.
His understanding of diabetes left him unable to make appropriate health choices and his daughter lived far away and only saw him on occasion. He ate TV dinners and found preparing food for himself an insurmountable challenge. His poor adherence was due to low “Health Capital” or “Health Literacy”, and his resource network wasn’t able to compensate.
Incorporating Patient Behavior into Clinical Guidelines
In clinical treatment guidelines, this is phrased as “Social Determinants of Health” which is presumed to account for 50% of health outcomes. SDoH are equally deemed by the American Heart Association to constitute a major impediment for delivering upon their ambitions of “Goals: to improve cardiovascular health of all Americans by 20% while reducing deaths from CVD and stroke by 20%.”
In clinical trials, we excel in quantifying the biometrics that constitute predictors of poor health outcomes. Zooming in on the cardiometabolic space, we are becoming increasingly diligent in describing the causalities of dysglycemia, dyslipidemia, other markers of atherosclerosis, aspects of hypertension, fatty liver parameters etc. toward adverse Health Outcomes. These are important additions to our scientific understanding of the diseases that together characterize the “Cardiometabolic Pandemic” we see unraveling across the globe with more than 650 million people being obese and 530 million people (10.5% of the world’s population) living with Type 2 diabetes.
In the “non-health related” part of our lives, social media, mainstream media, streaming services, and other outlets have used complex algorithms for years. These approaches have been used to understand consumer preference, direct advertising, and influences on lifestyle behavior, with very high levels of acceptance. For the pharmaceutical industry and investigators, the question now beckons: are there validated methods for how similar approaches can be applied in clinical research to quantify and modify behavioral and psychological factors to augment treatment efficacy and weight loss? And subsequently, is it possible to include a behavioral modification tool to our clinical trial platforms to ensure lifestyle standardization throughout the study? Once the data is available on an individual trial basis it may be possible to obtain a marketing license for a standalone or companion digital therapeutic.
We Suggest the Following General Approaches to Clinical Trial Design:
First, in the context of a clinical trial for diabetes or obesity we need to adhere to FDA recommendations to standardize lifestyle modification – Guidance for Industry Developing Products for Weight Management (fda.gov). But we can take this further by really trying to understand the individual patient and ensuring they get the best possible outcome from the drug and treatment advice.
Second, we can quantify the patient’s personality, mood, and health behavior by screening at baseline. This is important as personality is a known determinant of nonspecific outcomes such as adherence in clinical trials.
Third, we propose using validated behavioral digital tools that are based on principles of cognitive behavioral therapy. This will create a dataset that can characterize and describe these in contexts with the biometrics.
Fourth, we recommend a feedback loop to the patient. We advocate the use of validated behavioral modification modules combined with machine learning. This can provide real-time feedback to clinical trial participants, with the goal of modifying their current behaviors in real time.
Translating General Recommendations to Specific Actions
All of this needs to happen with patient centricity in mind and without increasing the patient burden of a clinical trial. This can only happen if the insights are captured in a seamless site and patient experience solution.
At the patient level this approach will improve the likelihood of therapeutic effectiveness and ensure that activity levels, food intake, and glucose levels are aligned with a putative lifestyle standard for weight loss. Let’s apply digital health technologies in addressing the health inequities of cardio metabolic diseases. After all, it’s not the efficacy obtained in a clinical trial that delivers the promise of a drug, it’s the effectiveness of it observed in the real world. We believe that technology holds the promise of mitigating this gap if we are sufficiently curious to understand how to do it.
The question is how to take a structured approach to capture and characterize behavior and potentially provide “cognitive behavioral therapy” to modify behavior.
Our Specific Solutions to Capture and Assess Behavior are as Follows:
Clinical ink excels in capturing data directly from patients in clinical trials in a patient-centric manner. We now have an important opportunity to gain insights beyond capture of endpoints into behavioral aspects impacting clinical trial protocols. If patients are unable to adhere to the drug treatments or follow trial protocol procedures, neither they nor the sponsors will have the insights needed to thoroughly evaluate new treatment options for “people like them”, and the patients in the trial will have to be replaced, adding cost and time.
We have thus partnered with Observia, the developer of SPUR™️, and together we are for the first time bringing a clinically validated digital behavioral assessment into clinical trials for drug development programs.
SPUR™️ stands for “Social, Psychological, Usage, and Rational”, the four overarching dimensions categorizing the drivers of patients’ decision-making processes. SPUR™ is a dynamic and predictive assessment tool that integrates decades of proven behavioral science into a validated digital solution for detecting a patient’s risk of non-adherence and the drivers of their health behavior.
In our integrated solution, investigators are provided with insights into risk of non-adherence as well as specific underlying drivers of the risk. For example, two people could both present the same high risk of non-adherence, but for one, that might be because they have limited understanding of the benefits of the proposed treatment coupled with a difficult family situation whereas the other person’s risk is primarily driven by more practical difficulties.
Conclusion
Every individual has a unique personality. The best way to ensure their successful participation in a clinical trial is to formally assess their behavior and risk of adherence, and ensure they have the right support.
Having these insights available in real time will provide clinical trial investigators with objective and validated points to consider in the enrollment of patients, and adequately manage the risk of dropout and adherence to drug and protocol.
Authors
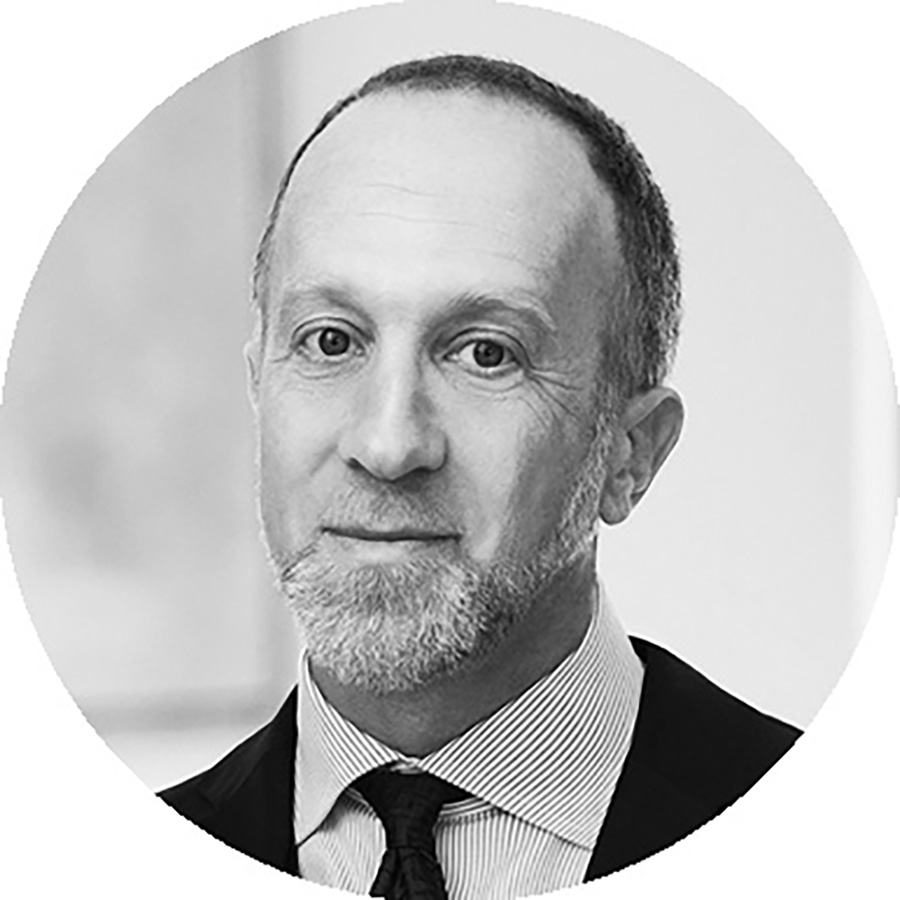
Jonathan Goldman, M.D.
CEO
Clinical ink
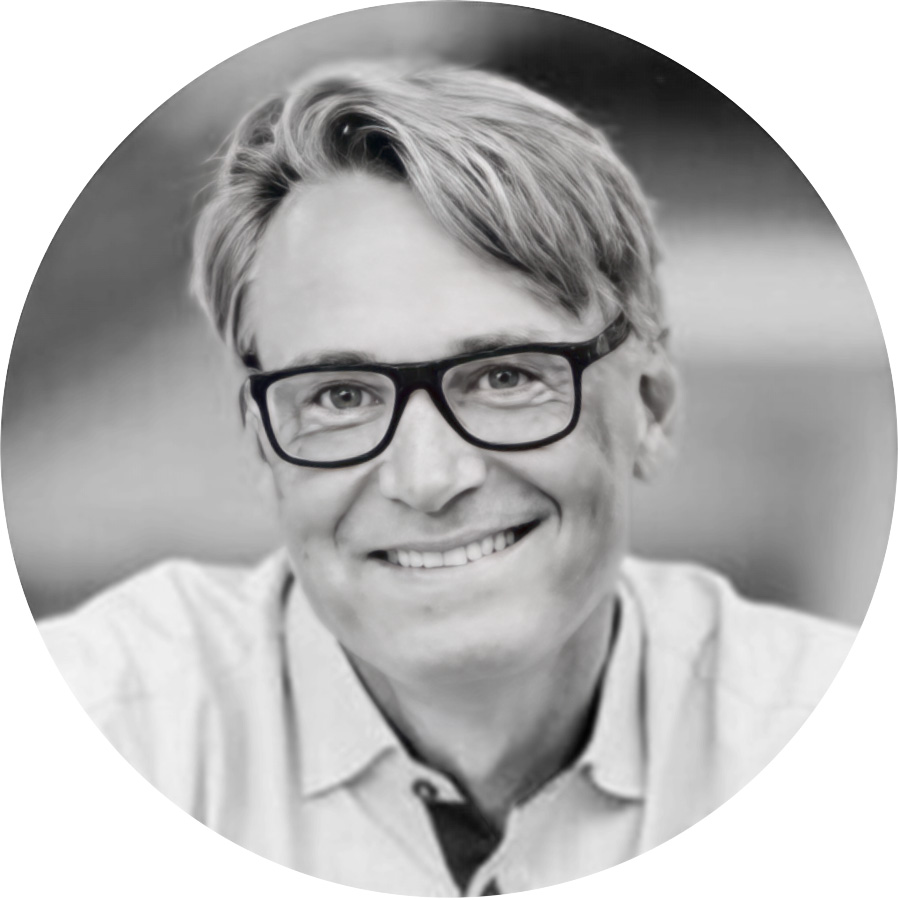
Christian Born Djurhuus, M.D., PhD, BSc.
Diabetes Advisor to Clinical ink
Owner of Djurhuus Consulting