An Engineering Feat that Requires an Expert, Multidisciplinary Team
Years ago, the US Bureau of Product Safety within the Food and Drug Administration (FDA) required that an ad demonstrating the flexibility of a razor blade include the warning: “Don’t try this at home.” These are wise words that can also be applied to sensor fusion, or fusing together biometric data from multiple consumer-grade sensors and wearables, along with a variety of other data sources. The fused data reveal rich details about a patient’s condition and experience and can be used as secondary endpoints in drug development.
The process of developing or adapting sensor/wearable applications to collect digital biomarkers is a very involved – and highly specialized – one that requires a mix of engineering, computer science, and, of course, clinical knowledge. Ensuring success in such an effort requires:
- An interdisciplinary team. Collectively, those working to fuse multiple real-world data inputs must understand digital technologies, data analysis methodologies, and clinical trial processes. As a start, they must be familiar with the different device operating systems and applications and what they are capable of capturing. They must also be experts in designing user experiences, in cognitive psychology, and signal processing. At the same time, the team must know how the data will be used to support drug development – which means understanding the characteristics of the particular disease and the relevant ways that disease progression and disease burden are measured. This is all in addition to needing to know how to work with all the moving pieces of a clinical trial, which includes satisfying the information needs of Institutional Review Boards (IRBs) and regulators.
- Data science expertise across modalities. The data collected from different types of digital devices are so unalike that they require the expertise of very specialized data scientists. For instance, analyzing voice data requires different expertise than analyzing movement data, and it is unlikely that the same data scientist could do both.
- A flexible data analysis platform. The technology must be able to ingest diverse types of data (including from third-party applications) and support multivariate analyses, modeling, and simulation through machine learning and artificial intelligence.
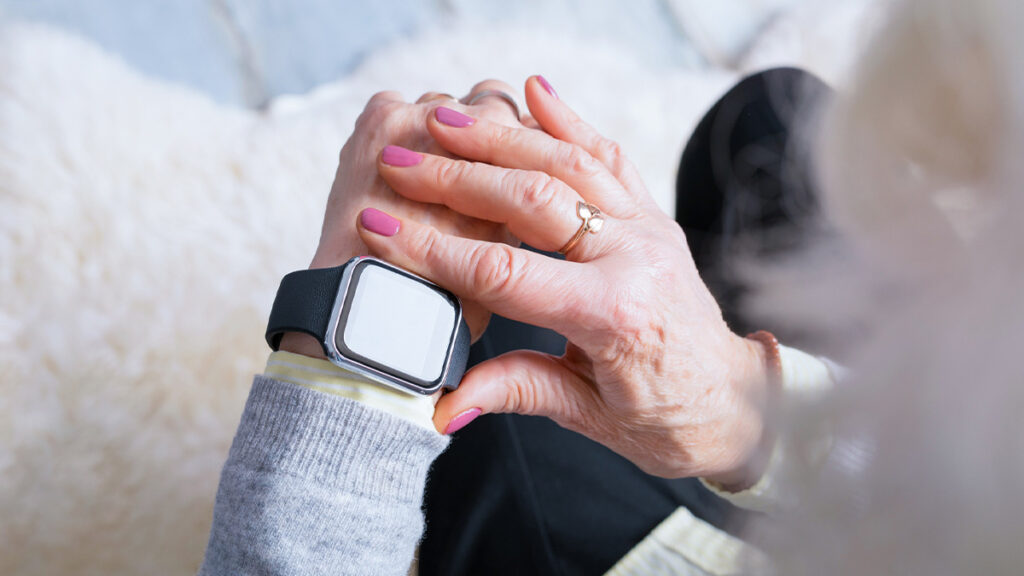
The goal – meeting data capture and reporting needs – by fusing digital biomarker data with other data may sound standard enough for the industry, but in actuality it is quite a new field that few have mastered to date. And, knowing how to collect and work with data from one type of device doesn’t translate into experience that can apply to any other type of device, let alone to fusing different data sets. Those who “try it at home” so to speak and attempt to implement sensors and wearables without a fusion strategy and the necessary experience and expertise are unlikely to be successful.
Want to understand the basics of sensor fusion? See our previous blog post.
Learn more about Sensor Fusion in the White Paper “Digital Biomarkers as Endpoints in Parkinson’s Disease”
See a real-world example of Sensor Fusion in action in our WATCH-PD Case Study.
Author
Joan Severson
Chief Innovation Officer,
Clinical ink